June Featured Project | Improving Additive Manufacturing using Machine Learning
June 8, 2022 | By Ylan Phan and Jenna Ross

Additive manufacturing is, as the name suggests, the process of creating an object by building layer by layer. It is considered among the most disruptive technologies of our age and is predicted to be at the forefront of the fourth industrial revolution. Virtually every industry has adopted Additive Manufacturing (AM) technologies, including automotive, product development, medical, aerospace, electronics, and more. It is estimated that 47% of manufactures use additive technology.
Innovation in this space is occurring at a fast rate due to AM’s potential to be the most effective solution to various pain points in manufacturing. The main draw of AM is that it produces drastically shorter lead times compared to conventional processes and supports rapid prototyping, thus increasing speed to market for products. In addition, it allows for easy manufacturing of objects with complex geometry. Yet, due to the lack of established qualification methods, especially due to a scarcity of online process monitoring and control methods, AM technologies are immature, causing uncertainties and increased risks for involved stakeholders. For AM parts to be more broadly used, more information is needed about the 3D printed part: Is the part within required manufacturing tolerances? How strong is the material? How might the part fatigue or fail?
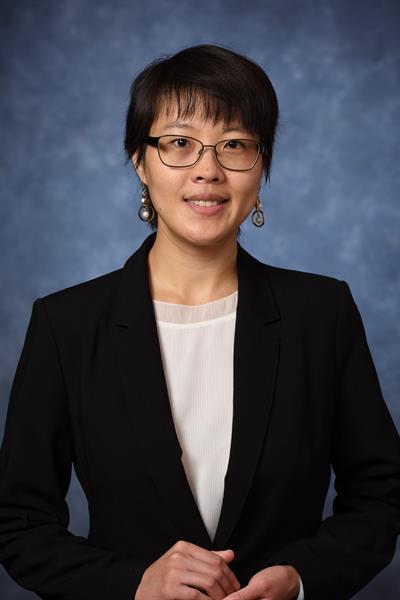
This is where Dr. Xiayun Zhao and her team of researchers come in. Her MDS-Rely project, “Achieving Reliable Laser Powder Bed Fusion Based Additive Manufacturing via Machine Learning of In-Situ Optical Profilometry Monitoring” is focused on improving the qualification and understanding of metal parts that have been created by laser powder bed fusion (LPBF) printing. LBPF is a type of AM where thin beds or layers of powders are applied to the part and a laser is used to sinter the powder at specific locations.
Her group integrates fringe projection profilometry (FPP) with LBPF so that important layer-wise surface topography information of the printed geometry can be obtained in real-time. This information can be used to qualify the printed part’s surface property or for real-time feedback control. Additionally, their project intends to discover the relationship between the printed material’s induced material defects, surface topography, and mechanical properties.
“We implement machine learning, a data driven technique, to improve the overall accuracy and applicability of traditional FPP into LPBF part qualification and process-structure-property correlation analysis”
-Haolin Zhang
Haolin Zhang, a PhD student working in Dr. Zhao’s lab, gave us more insight into the value of their research, “We implement machine learning, a data driven technique, to improve the overall accuracy and applicability of traditional FPP into LPBF part qualification and process-structure-property correlation analysis. The comprehensive machine learning based model will be constructed to correlate printed part structure, process defects, and the surface topography acquired by the fringe projection profilometry.”
Dr. Zhao’s research contributes to the MDS-Rely center’s mission to “apply data science-informed research to better understand the reliability and lifetime of essential materials.” She envisions a tool that can help with decision making and control input computing to optimize or control their LPBF machines for printing quality parts at reduced cost or waste.