PROJECT INFORMATION
Project Presentation Details
Effects of Aerosol Jet Printing Parameters on the Lifetime Performance of Additively-Manufactured Flexible Circuits
Biomedical devices such as implantable electronics and flexible sensors can be enhanced through the use of Aerosol Jet Printing (AJP) as a micro fabrication technique. A more affordable and customizable alternative to traditional methods, AJP allows for microgabrication outside of a clean room. However, there is limited research regarding its efficacy. Dr. Gbur, Mitchell Melander, a MS student, and their team aim to fill this gap by studying the qualitative and quantitative characteristics of AJP flexible sensors and the morphology of their conductive traces. Successful prints are studied through extensive mechanical and electrical testing to ensure that the ink’s adhesion to the substrate can withstand bending forces without impacting its electrical resistance. From their data collection, the team intends to develop processing maps that correlate to printing parameters in order to create a guide for future development of flexible sensors.
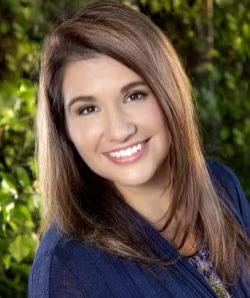
Janet Gbur
Assistant Professor
Case Western Reserve University
Non-Invasive Detection of Defects during Coatings Manufacturing
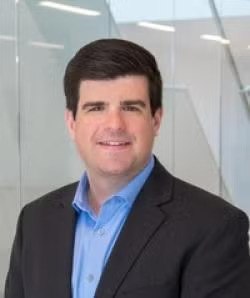
Chris Wirth
Associate Professor
Case Western Reserve University
Beyond negatively impacting the aesthetics of consumer products, defects such as bubbling, sag, pinholes, and streaks, which occur during coating manufacturing, can impair a product’s performance and cause premature failure. Dr. Chris Wirth and Dr. Sithara Vinod aim to utilize non-invasive image processing techniques to detect said defects, determine the mechanism of their cause, and improve product performance.
Through imaging modalities such as epifluorescence, bright field microscopy and Optical Coherence Tomography, the team is developing image processing algorithms to detect defect formation in coatings undergoing solvent evaporation. In particular, Dr. Wirth is exploring the ‘orange peel’ effect which results from Marangoni instabilities caused by convection cells. Through their experimentation, the team strives to develop a detection technology that can be employed in automated coating manufacturing environments.
Predictive Framework to Indicate the Age of Plastics for Proper Recycling
Plastic generation has risen by more than 900% since the 1980s and its product often sits in landfills for up to several decades. Dr. Metin Karayilan, Dr. Divita Mathur, and Dr. Sanmukh Kuppannagari are interested in mitigating this issue by developing a method to determine the plastics’ age in order to enhance the efficacy of recycling procedures. Through experimentation, the team is studying the spectral properties of blend material to predict aging processes such as heat, chemicals or irradiation to measure the absorbance transmittance and NMR spectra. From the spectral data, the team is utilizing artificial neural networks for age prediction to then be co-optimized by measuring mechanical properties. Their goal is to develop a translatable framework for industrial materials that require age determination, demonstrate the framework in industry-relevant additives, and diminish the plastic pollution crisis.
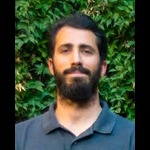
Metin Karayilan
Assistant Professor
Case Western Reserve University
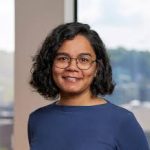
Divita Mathur
Assistant Professor
Case Western Reserve University
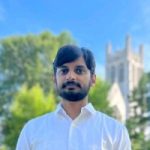
Sanmukh Kuppannagari
Assistant Professor
Case Western Reserve University
Machine Learning Methods for Optimizing and Innovating Structural Color Paints and Coatings
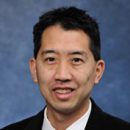
Paul Leu
Associate Professor
University of Pittsburgh
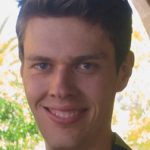
Oliver Hinder
Assistant Professor
University of Pittsburgh
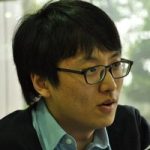
Jungtaek Kim
Post-Doc Researcher
University of Pittsburgh
Pigments are utilized in a variety of industries such as art, fashion, food, construction and automotive. However, traditional pigmentation is chemically derived which can cause negative environmental impacts and fade over time. On the other hand, structural colors which are bio-inspired by butterflies, peacocks, and beetles, can produce a gamut of vibrant colors that are both durable and environmentally friendly. Dr. Paul W. Leu, Dr. Oliver Hinder, and Dr. Jungtaek Kim aim to create a comprehensive database detailing a variety of these structures and the colors they produce by developing a machine learning framework for structural color paints and coatings. With said ML models, they intend to fabricate layer-by-layer coatings and color swatches that can be utilized to determine color durability through tests such as abrasion, 85/85, and UV degradation.
Enhancing Battery Degradation Analysis and Thermal Runaway Prediction
This project focuses on thermal runaway (TR), a major safety risk in lithium-ion batteries where self-heating can lead to fire, explosion, or gas release. While relatively rare in single cells, the risk increases significantly in systems with many cells, making TR a critical issue in modern electronics and vehicles.
Dr. Iyengar and his team are collecting data from experiments, incident reports, and related phenomena to identify early indicators of TR. They are applying ARIMA models for stationary voltage and temperature data, and nonlinear approaches such as splines and neural networks for highly nonstationary periods. A known PDE model for temperature is incorporated with noise to explore TR behavior in a stochastic setting. This work aims to improve prediction accuracy, generate simulation data, and provide insights into the mechanisms underlying thermal runaway.
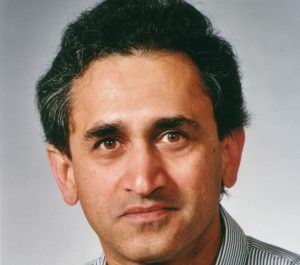
Satish Iyengar
Professor and Associate Chair
University of Pittsburgh
LitDB
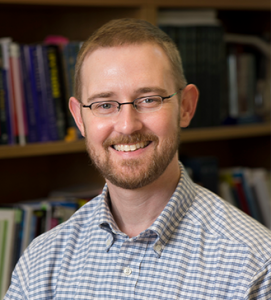
John Kitchin
Professor
Carnegie Mellon University
Dr. John Kitchin created LitDB, a tool that allows users to sift through and curate custom local databases of scientific literature focused on their user-specified topic. Inspired by the sheer amount of scholarly resources being made available online every day and the time it takes to explore them, LitDB provides a simple and easy-to-use alternative to traditional online research by optimizing the research process with a customizable database.
LitDB, which can be found on Dr. Kitchin’s GitHub, allows users to supply a research paper as a jumping-off point. From it, the tool compiles a local database of the titles and abstracts of each reference, citing source, and related source tied to the original paper using data from OpenAlex and Turso. Users can then query the database with simple terms, receiving the most relevant sources along with their relativity score. The tool also features vector search, enabling users to query with longer natural language phrases and make deeper connections beyond simple matched terms.
If users are not satisfied with the similarity score between their query and the supplied articles, they can expand the database by collecting references, citing sources, and related sources from the top three articles. This iterative process grows the database, increasing the likelihood of discovering more relevant papers.
Quantitative Characterization of Chemical Interaction of Solutes with Defects for Predicting Intergranular Corrosion
Dr. Hyeji Im and their team are investigating the potential of laser additive manufacturing (AM) to develop molybdenum alloys as fuel cladding materials with enhanced corrosion resistance. This research has two main aims: (1) explore the potential of laser AM as a viable processing route to develop molybdenum alloy as a fuel cladding material, and (2) generate quantitation input data on thermodynamic and diffusional interactions between defects and solutes, which can serve as a guide for predicting intergranular corrosion.
By introducing silicon (Si) and boron (B) into Mo grain boundaries, the team aims to improve cohesion and mitigate impurity segregation, strengthening the material against intergranular failure. To further enhance corrosion resistance, the researchers are employing a novel laser exposure technique to introduce surface defects, promoting the rapid formation of protective oxide layers. Additionally, they are generating precise datasets on defect-solute interactions for machine learning models, which can predict intergranular corrosion and inform material design strategies. This research has the potential to extend the lifetime of nuclear materials and improve reactor resilience under extreme conditions.
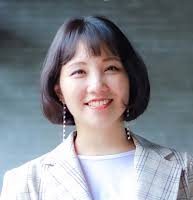
Hyeji Im
Assistant Professor
Case Western Reserve University
Laser Powder Bed Fusion Surface Defects Control via In-situ Topography Feedback and Multimodal Monitoring-Guided Laser Rescanning
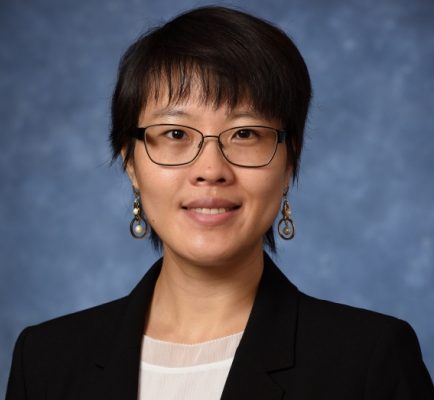
Xiayun Zhao
Assistant Professor
University of Pittsburgh
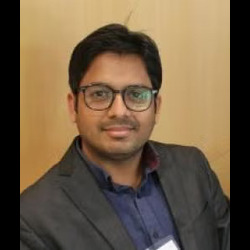
Pawan Tripathi
Assistant Professor
Case Western Reserve University
This project aims to improve the quality and reliability of laser powder bed fusion (LPBF) by integrating in-situ surface topography feedback and multimodal monitoring systems. It focuses on two main objectives: (1) identifying and analyzing critical surface defects such as spatter and roughness using fringe projection profilometry (FPP) and deep learning, and (2) developing optimized laser rescanning strategies guided by real-time monitoring of melt pool dynamics and surface features. By combining PI Zhao’s in-situ imaging systems with PI Tripathi’s multimodal data analytics, the team will establish a data-driven framework for adaptive process control and defect mitigation in LPBF.